Video Analytics Solution to Uncover Powerful Business Insights
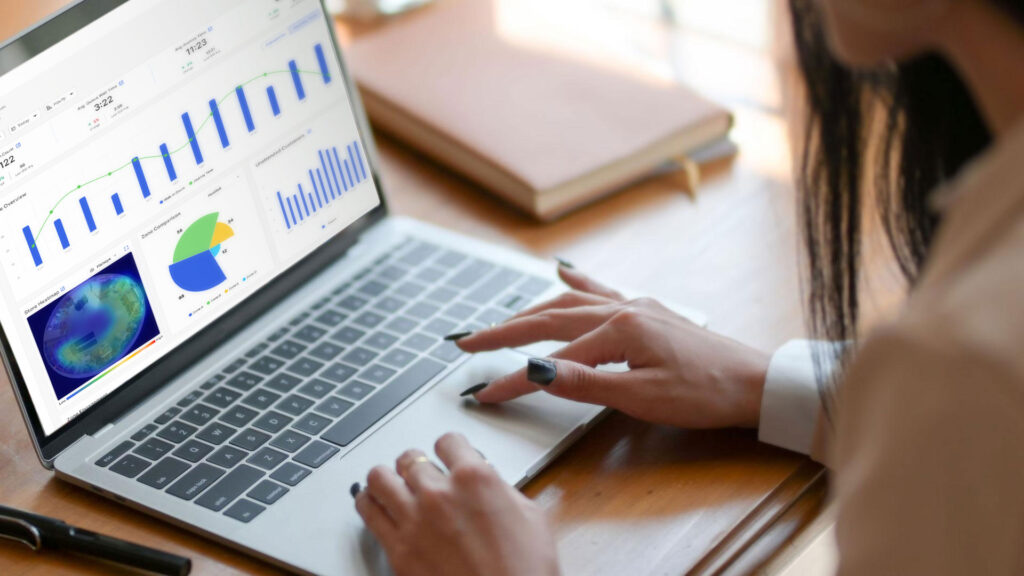
How Video Analytics Can Transform Operations
Video analytics offer significant opportunities for retailers, restaurants, and other consumer-facing businesses to enhance revenues, reduce costs and improve loyalty and efficiency.
Optimize Drive-Thru Operations
Monitor and streamline drive-thru service. Track vehicle queue lengths, service times, and order accuracy to enhance efficiency and customer satisfaction.
Analyze Customer Footfall
Track and analyze customer movement patterns within a store to optimize product placement and store layout. Helps increase sales by strategically positioning high-demand items.
Optimize Queue Management
Analyze queue lengths and wait times at the checkout counters to deploy staff efficiently, reducing customer wait times. Provides data-driven insights to manage peak hours better and improve customer experience.
Analyze Customer Interactions
Analyze employee behavior and customer interactions at the POS and other areas to identify training needs and improve staff performance. Provides actionable insights for targeted training programs, enhancing overall productivity.
Improve Merchandising
Understand how customers interact with shelves, display units, and kiosks. Perform inventory audits remotely, review instances of empty shelves or how fast products find their way to displays. Additionally, A/B test every display with department-level traffic counting and customer analytics.
Monitor Health & Safety Compliance
Automatically track and ensure cleanliness protocols are followed in real-time and employees wear masks, gloves, and hairnets. Alert supervisors if cleaning schedules are missed or protocols aren’t followed.
Enhance Loss Prevention
Identify and respond to suspicious activities in real time, reducing theft and loss. Detailed analytics with video help security teams investigate incidents more effectively.
Measure Marketing Effectiveness
Find out how online marketing campaigns translate into customer traffic at stores. Compare traffic across stores and leverage traffic insights to accurately attribute sales conversions to campaigns.
Watch How Video Analytics Can Transform Retail and Restaurant Operations
Designed for Multi-Location Enterprises
Open Architecture
- Our video analytics solution is compatible with any ONVIF-compliant camera and those that support RTSP streaming.
- Our solution works with most IP cameras, and smart cameras with built-in computing capability and memory.
Resilient Connectivity
- Our video analytics solution is designed to work with limited bandwidth and be completely offline without losing data.
- Data captured when the network is unavailable will be instantly pushed to the cloud once the connectivity is restored.
Drill-Down Reporting and Audits
- Enterprises can easily compare store performance by location across all operational areas and filter the data to a specific time of the day.
- Users can annotate images and share them securely internally for compliance training or perform an audit of the analytics data by quickly locating the relevant recordings.
Well-Documented API
- Pulling data into custom dashboards or any other application for analysis can be easily implemented using a secure, read-only REST API in CSV or JSON format.
- Our video analytics API complies with SemVer standards for seamless change management and provides additional insights such as the health of the network and cameras.
Latest Updates and Insights
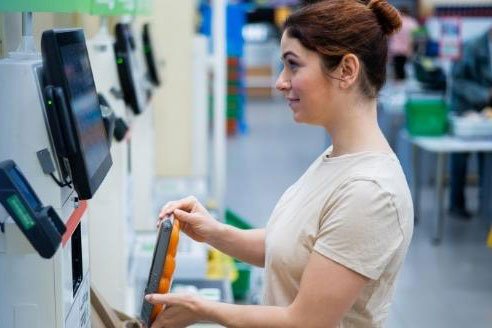
6 Game-Changing Retail Technology Trends in 2024
Retail technology trends in 2024 – Contactless Stores, AI-Enabled Security Cameras, Retail Video Analytics, Metaverse, Retail Demand Planning.
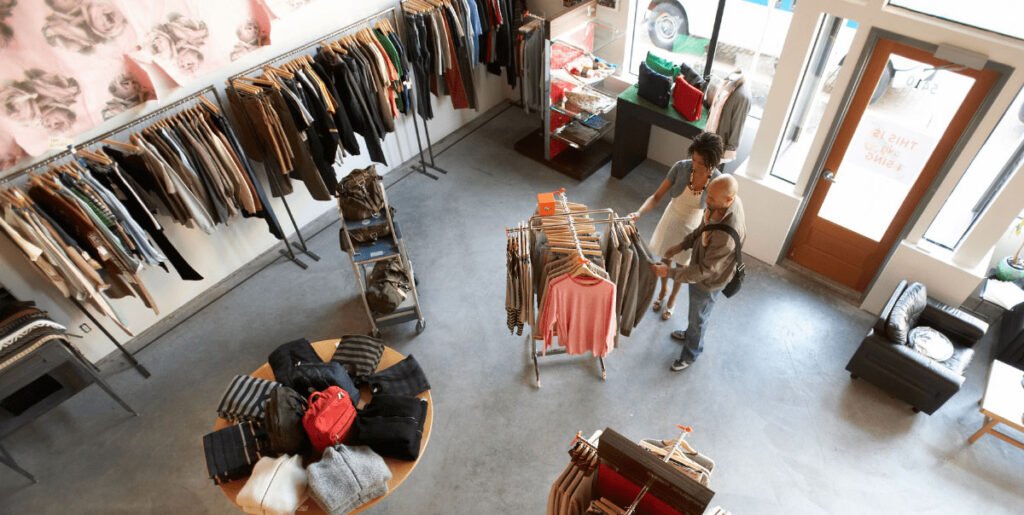
Retail Store Layout Optimization with Video Analytics
Get actionable tips to leverage video analytics for retail store layout optimization to improve customer satisfaction, sales, and compliance.
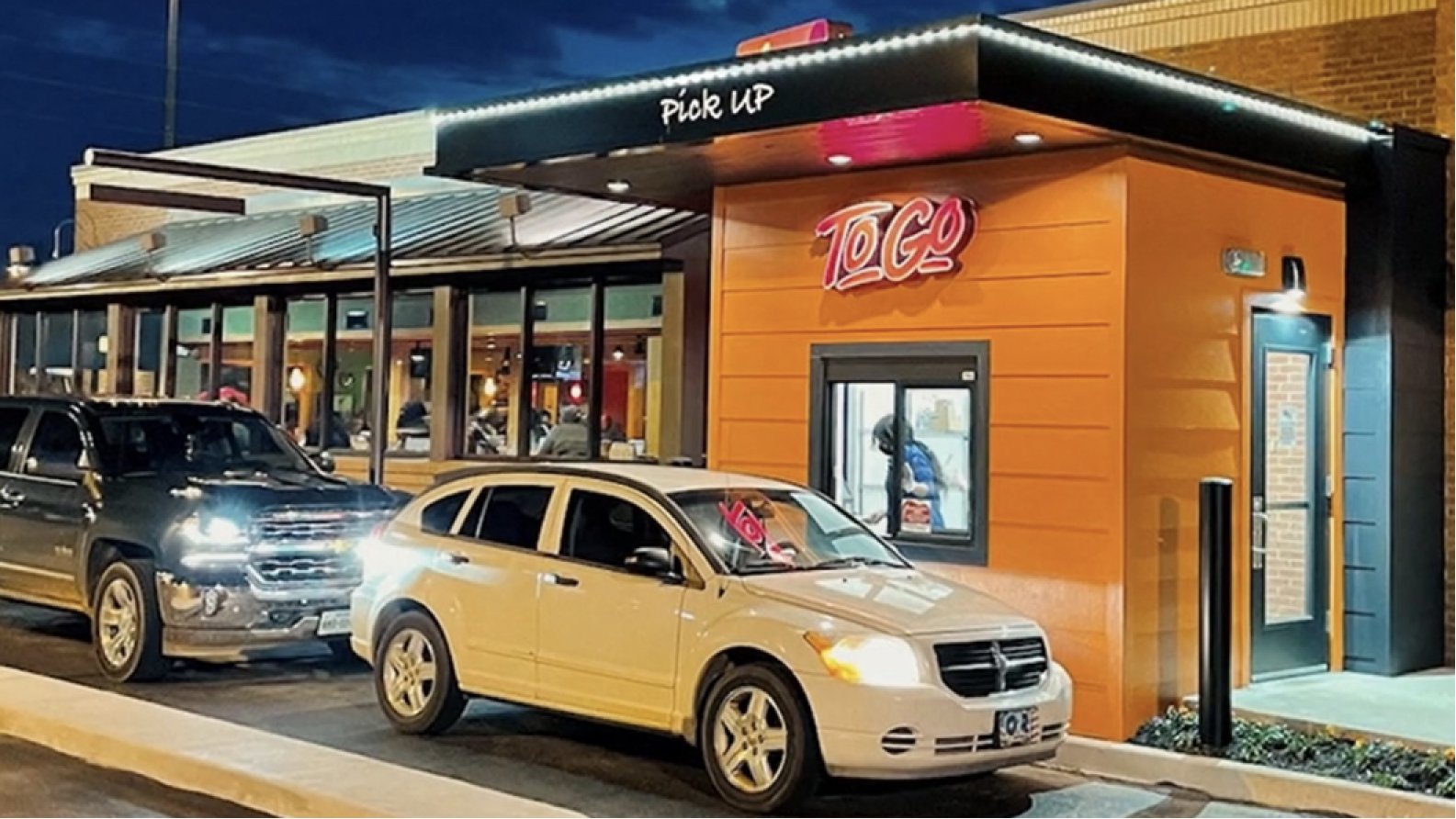
7 Groundbreaking Drive-Thru Concepts and Trends for QSRs in 2024
Go Beyond Surveillance with Interface Video Analytics Solution
Frequently Asked Questions
Video analytics is a process that involves analyzing video data to extract insights, patterns, and meaningful information using computer algorithms and machine learning techniques. It can be used for various purposes, such as security, marketing, and operations.
Video analytics systems typically use cameras or sensors to capture video footage and then process the data to detect, classify, and track objects or events of interest. This can include people, vehicles, objects, or specific behaviors.
The analysis of video data can provide businesses with valuable insights into customer behavior, sales trends, operational efficiency, and security risks. For example, retailers can use video analytics to measure foot traffic, optimize store layouts, and monitor inventory levels. Security teams can use it to detect potential threats, identify suspicious activity, and respond quickly to incidents.
Video analytics systems may use a range of technologies, such as deep learning, computer vision, and artificial intelligence, to analyze video data. The software may be installed on the camera itself or run on a separate computer or server. Some systems may also use cloud-based analytics to enable real-time monitoring and alerts.
Video analytics and traditional video surveillance are similar in that they both involve the use of cameras to capture video footage. However, there are some key differences between the two:
- Purpose: The purpose of traditional video surveillance is primarily to record and monitor video footage for security purposes, such as detecting and preventing theft, vandalism, or other criminal activity. Video analytics, on the other hand, is designed to analyze and extract insights from video data, with the goal of improving business operations or customer experience, in addition to enhancing security.
- Automated analysis: Unlike traditional video surveillance, which relies on human operators to monitor and analyze the video footage, video analytics is often automated, using advanced algorithms and machine learning techniques to analyze the video data in real time.
- Customization: Video analytics can be customized to suit a wide range of applications and use cases, allowing organizations to extract insights and value from their video data in unique and specific ways. Traditional video surveillance, on the other hand, is generally more limited in its capabilities and applications.
- Scalability: Video analytics is designed to be highly scalable, with the ability to analyze large amounts of video data in real time. This makes it well-suited for organizations with large and complex operations, such as retail or restaurant chains. Traditional video surveillance, on the other hand, can be more difficult to scale up to handle large volumes of video data as it requires trained teams.
- Cameras or sensors: Depending on the application, different types of cameras or sensors may be used, such as IP cameras, thermal cameras, or RF sensors.
- Network infrastructure: Video data is often transmitted over a network, so a robust and reliable network infrastructure is critical. This includes switches, routers, and cabling that can handle the bandwidth and latency requirements of video data.
- Video management system: A video management system (VMS) is used to manage the cameras and video data. This includes functions such as video recording, storage, and retrieval. The VMS may also provide analytics capabilities or integrate with a separate analytics platform.
- Analytics software: Analytics software is used to analyze video data and extract meaningful insights. This may include software for object detection, facial recognition, or behavioral analysis.
- Storage infrastructure: Video data can take up a lot of storage space. Store infrastructure can include local storage on the VMS server or cloud-based storage.
Artificial intelligence (AI) enables video analytics systems to analyze large amounts of video data quickly and accurately, making it possible to detect patterns, identify objects, and extract insights in real time.
Here are some of the ways that AI is used in video analytics:
- Object detection and recognition: AI algorithms can be trained to recognize specific objects or people in video footage. This can include identifying faces, license plates, or specific items.
- Behavioral analysis: AI can also be used to analyze the behavior of people or objects in video footage. This can include tracking movement patterns, identifying abnormal behavior, or detecting suspicious activity.
- Predictive analytics: AI algorithms can use historical data to predict future events or behavior. For example, video analytics can be used to predict the likelihood of a security incident or to forecast customer traffic patterns in a store.
- Deep learning: Deep learning is a type of AI that can be used to analyze complex patterns in video data. It involves training neural networks to recognize specific patterns or features in video footage, such as facial expressions or product placement.
- Real-time processing: AI can enable video analytics systems to process and analyze video data in real time, allowing for immediate detection and response to events or behavior.
The accuracy of video analytics can vary depending on a number of factors, such as the quality of the video data, the complexity of the analytics algorithms, and the environmental conditions. However, video analytics can be highly accurate and reliable when implemented properly.
One of the key factors in achieving accuracy is ensuring that the video data being analyzed is of high quality. This means that the cameras or sensors used to capture the footage must be positioned and configured correctly and that the video data must be transmitted and stored to preserve its quality. In addition, the analytics algorithms used must be well-designed and optimized for the specific application.
Another important factor in achieving accuracy is ensuring that the video analytics system is properly calibrated and validated. This involves testing the system under a variety of conditions to ensure that it is able to accurately detect and analyze the events or behavior of interest.
Overall, the accuracy of video analytics can range from very high to somewhat lower depending on the specific implementation and application. However, with proper attention to factors such as data quality, algorithm design, and system calibration, it is possible to achieve highly accurate and reliable results.
Here are some of the challenges that may arise when implementing video analytics:
- Data quality: Video analytics requires high-quality video data to produce accurate and reliable results. Ensuring that the cameras or sensors used to capture the video are positioned and configured correctly and that the video data is transmitted and stored in a way that preserves its quality can be a challenge.
- Algorithm design: The design and optimization of the algorithms used to analyze the video data can be a complex and time-consuming process. Ensuring the algorithms are well-designed and optimized for the specific application is crucial to achieving accurate results.
- System integration: Video analytics systems may need to be integrated with other systems or applications, such as POS or intrusion alarms. Ensuring that the video analytics system can communicate effectively with other systems can be a challenge.
- Privacy concerns: Video analytics can raise privacy concerns, particularly if the system is used to monitor people or capture sensitive information. Organizations must ensure that they have appropriate policies and procedures in place to protect privacy.
- Maintenance: Video analytics systems require ongoing maintenance and updates to ensure they function properly.
Related Services